A Structured Approach To Machine Learning Life Cycle: A Case Study
LAU Byblos Campus - Block A Building, Room 710 & Online via Webex
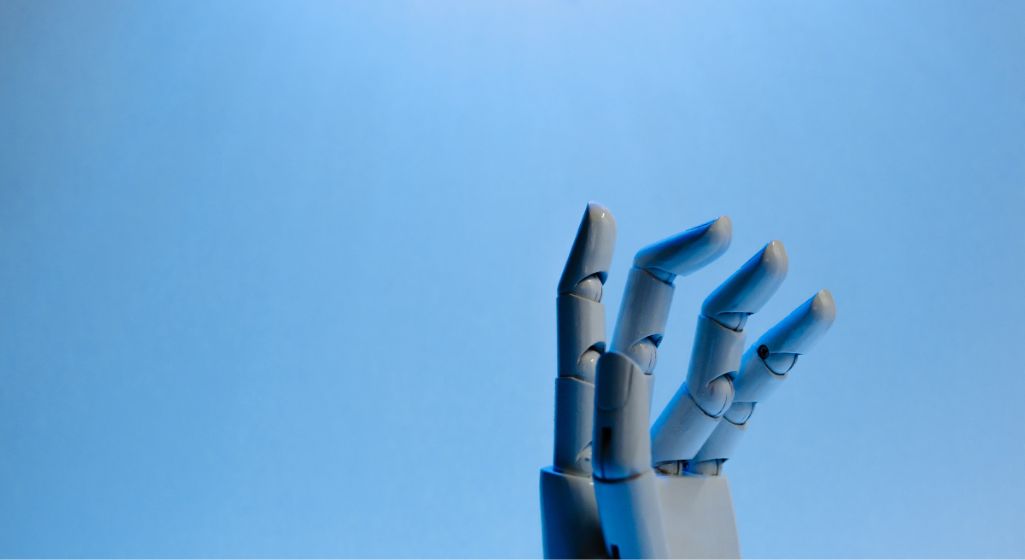
The LAU School of Arts and Sciences invites you to the talk event
A Structured Approach To Machine Learning Life Cycle: A Case Study
as part of its Research Seminar Series
About the speaker
——————————————
Dr. Edmond Abrahamian
Lead Data Scientist
Dr. Edmond is a lead data scientist at AT&T in Palo Alto, California. His current projects include real-time machine learning models to detect fraud in AT&T retail and small business sales channels. His other projects include anomaly detection of user inputs for fraud detection. In the past, he has worked on a variety of projects at AT&T including churn, location studies, network simulations, advertising, climate change and external affairs projects. Dr. Edmond started his career in cheminformatics, molecular modeling and drug design, where he specialized in data mining and pharmacophore perception.
He is a very avid racquetball player and also an avid cyclist.
About the event
——————————————
When it comes to comparing and deploying machine learning models, there are several considerations that can affect deployment decisions. Here, we describe our approach to a complete pipeline, from training to deployment. We share tips about how to compare models that were trained on the same (or different) dataset, as well as compare models that share the same (or different) features. We discuss how to “proof” a model prior to deploying it in production to make sure it will perform as expected. Finally, we share how we deploy models into production and what to watch for at the deployment level, and most importantly, how to debug anomalous model behavior.